Medical
- Home / Industries /
- Medical
Business intelligence in Medical
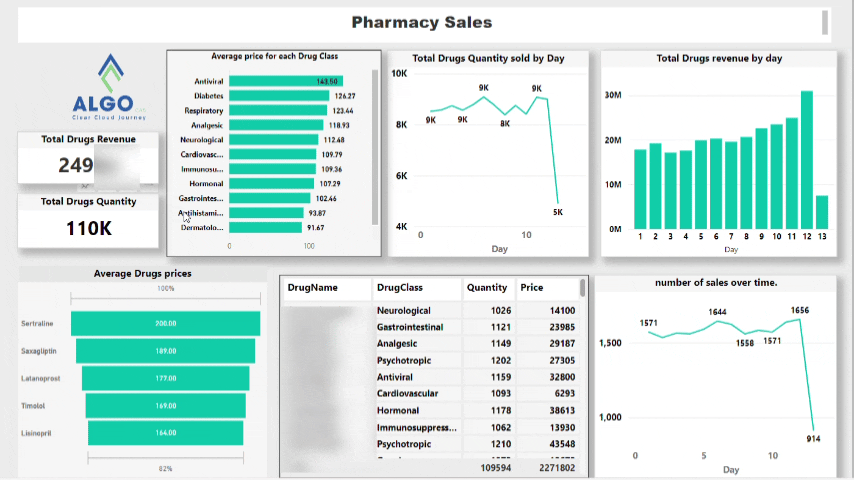
Smooth Operations: My dashboard keeps pharmacy operations running smoothly with updates on prescriptions, inventory, and patient engagement.
Smart Inventory: It helps us keep the right meds in stock, avoiding shortages and ensuring patients get what they need.
Better Patient Care: By tracking patient activity, we can provide better care and support, making sure they stay on top of their medications.
Financial Insights: It gives us a clear picture of our finances, helping us make smarter decisions to save money and boost profits.
Stay Compliant: With alerts for compliance issues, we can ensure we’re always following the rules and keeping everything above board.
AI in Medical
By analyzing digital images of bladder tumor tissue samples, an AI model assesses the chances of cancer spreading to nearby lymph nodes. This application requires a dataset of digital images of bladder tumor tissue samples along with corresponding information about the cancer spreading to nearby lymph nodes. The AI model processes these images using computer vision techniques and machine learning algorithms. It learns patterns and features from the images to predict the risk of cancer spreading to nearby lymph nodes based on the characteristics of the tumor tissue.
Using computer vision techniques, AI algorithms identify and segment blood vessels within medical images. This application relies on a dataset of medical images that include blood vessels. The AI model processes these images using computer vision algorithms to identify and segment the blood vessels from the surrounding tissues. It learns to distinguish the unique patterns and shapes associated with blood vessels, enabling accurate detection and segmentation.
AI algorithms analyze medical images of the brain to accurately locate and outline tumors. This application requires a dataset of brain images containing tumors. The AI model processes these images using deep learning algorithms and neural networks. It learns to analyze the distinct features and shapes of brain tumors, allowing it to accurately locate and segment them from the surrounding healthy tissue.
AI-based systems analyze medical images of breast tissue to detect and segment areas of concern. This application relies on a dataset of mammogram or breast ultrasound images. The AI model processes these images using convolutional neural networks (CNNs) and image processing techniques. It learns to identify patterns and abnormalities in breast tissue, enabling it to detect and segment regions of concern that may indicate the presence of breast cancer.
AI algorithms analyze eye images to predict the likelihood of developing cataracts. This application requires a dataset of eye images, including both healthy and cataract-affected eyes. The AI model processes these images using machine learning algorithms, specifically CNNs. It learns to analyze the characteristics of the eye lens and identify signs of cataract formation, enabling it to predict the likelihood of developing cataracts in the future.
AI algorithms analyze medical images to identify the presence of kidney stones. This application requires a dataset of medical images, such as CT scans or ultrasound images, that show the urinary tract. The AI model processes these images using image analysis techniques and machine learning algorithms. It learns to detect the presence of kidney stones based on their distinctive visual characteristics, helping doctors diagnose urinary tract disorders.
An AI-based diagnostics tool analyzes X-ray images to segment lung regions and detect conditions such as pneumothorax. This application requires a dataset of X-ray images along with corresponding diagnostic information. The AI model processes these images using deep learning algorithms and computer vision techniques. It learns to segment lung regions, detect abnormalities like pneumothorax, and calculate disease probabilities based on the patterns and features extracted from the X-ray images.
AI utilizes historical data and advanced forecasting techniques to predict monthly sales for various drugs. This application requires historical sales data for different drugs. The AI model processes this data using statistical analysis and predictive modelling techniques. It learns the patterns and trends in drug sales over time, allowing it to forecast future sales on a monthly basis.
AI algorithms utilize historical data and patterns to predict the quantity of drugs that will be needed in the future. This application relies on historical data of drug sales and usage. The AI model processes this data using time-series analysis and predictive modeling techniques. It learns the patterns and factors influencing drug quantity, allowing it to forecast the future demand for drugs accurately.
Leveraging the Full Potential of Your Cloud Investment
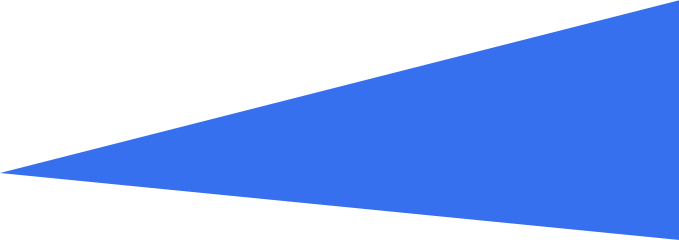